The Digital Twin Paradox: Can AI-Driven Efficiency Offset the Environmental Footprint of Building Operations?
- stauss5
- 3 days ago
- 5 min read
Buildings are among the world’s largest energy consumers, responsible for about 30% of global energy use and 26% of energy-related emissions. In the EU alone, buildings contribute 35% of energy emissions. These pressures call for urgent change, and digital twins (DTs) are emerging as a powerful solution. As DT technology enables real-time simulation and optimization across a building’s lifecycle, it holds significant potential to reduce emissions and energy use. The market reflects this promise, with DTs in buildings expected to grow from $3.6 billion in 2022 to $14.9 billion by 2027, at a CAGR of 32.8%. Furthermore, 75% of real estate companies view digitalization as essential, and 87% of executives believe AI can play a key role in addressing climate challenges.
With a digital twin, designers and engineers can perform thorough lifecycle analyses from the early design stages through to operation, optimizing both energy use and carbon footprint at every phase.
In the design phase, digital twins (DTs) enable architects and engineers to test scenarios and optimize for energy efficiency, potentially reducing carbon emissions by up to 50%. During construction, DTs help manage resources efficiently, cutting upstream emissions and reducing on-site waste by up to 25%.
With roughly 80% of a building's total lifecycle costs occurring in the operation phase, DTs offer significant value through real-time monitoring of factors like energy use, indoor air quality, and occupant behavior. DTs continuously analyze these aspects, identifying inefficiencies and providing actionable measures for improved energy management. In office buildings, for instance, AI-enabled DTs have demonstrated an impressive energy-saving potential of up to 37% by optimizing HVAC systems. While residential and educational buildings exhibit lower savings potential, at 23% and 21% respectively, the implementation of DTs still enhances overall efficiency.
While DTs can drive substantial energy efficiencies, there is a rising concern that the AI infrastructure supporting them may offset some of these benefits through its own resource demands.
AI development relies heavily on data centers, which are responsible for a rising share of global emissions, contributing to 2-3% of global emissions. In fact, Microsoft's CO2 emissions has risen nearly 30% since 2020 due to data center expansion, and Google’s emissions surged by nearly 50% from 2019 to 2023 as energy demands increased.
To get a feeling of the environmental footprint of AI training and inference, let’s examine some models in numbers, see Figure 1. Training multimodal models like GPT-3 generates 502 metric tons of CO₂, which is nearly 9 times the lifetime emissions of the average American car. A single ChatGPT query can consume up to 100 times more energy than a Google search query.
On the other hand, task-specific models like predictive analytics and reinforcement learning models, typically used in building systems, the energy demands remain relatively modest but still impactful. For instance, a small predictive model of about 5 million parameters requires 9.5 kWh of energy for training and 0.3 Wh per inference, amounting to around 4 kg of CO₂ emissions (assuming similar energy mix).
Scary? Alarming?
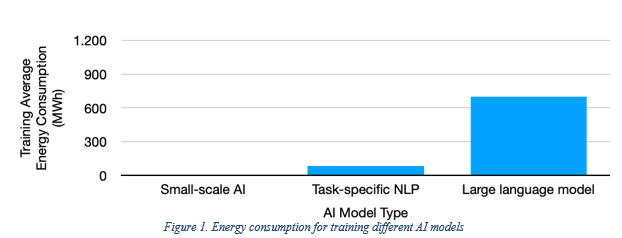
Figure 1. Energy consumption for training different AI models
While the environmental impact of training AI models for digital twins is a valid concern, it’s crucial to contextualize these emissions relative to the energy demands of building operations. While training requires energy, this one-time cost should be contrasted with the building’s emissions from operation and the ongoing energy savings enabled by the digital twins. For this comparison, let’s consider a range for annual energy consumption in commercial, residential and office buildings.

Figure 2. Range of annual energy consumption of different buildings
We see that small-scale AI models have negligible energy demands relative to the annual operations of even small residential buildings. Mid-scale task-specific NLP models, although more energy-intensive, have comparable energy use to large office buildings, while Large Language Models (e.g. GPT3) can require as much energy as a commercial building’s annual operation. This suggests that while AI model training requires a significant one-time investment, it can be reasonable when scaled against large, energy-intensive buildings or multiple smaller buildings.
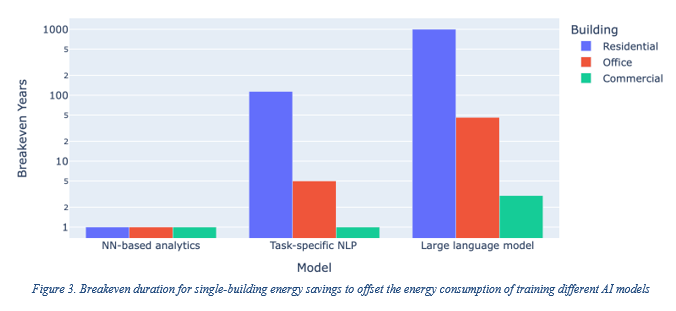
Figure 3. Breakeven duration for single-building energy savings to offset the energy consumption of training different AI models
Buildings optimized with AI-driven digital twins have the potential for significant annual energy savings — particularly in commercial and office settings. For residential buildings, these savings alone could offset the initial energy used for training small-scale models within the first year, while office and commercial buildings may offset even larger models' energy demands over a few years of operation. For high-energy-use buildings, this savings potential translates into a substantial environmental benefit. Typically, a single AI model is rolled out to multiple buildings, thus the collective energy savings is likely to accelerate the breakeven point.
Moreover, for building analytics and management applications, multimodal large models are rarely trained from scratch. Rather, pre-trained or fine-tuned models are used. While this doesn’t change the substantial energy consumed and the embodied emissions in the model’s training, it implies that minimal or no resources are additionally required to utilize such models in building applications.
While AI-driven savings contribute significantly, the wider framework of sustainable policies and energy sources is fundamental. The goal of achieving net-zero emissions can only be reached by combining AI-driven efficiency with strong energy policies, carbon pricing, and widespread use of renewable energy—both for IT infrastructure (e.g., data centers and AI infrastructure) and for the buildings sector itself. By pairing AI-driven DTs with low-carbon energy policies, we could cut energy consumption by 40% and reduce emissions by up to 90% by 2050.
Several measures and strategies have emerged to address AI’s energy and emissions concerns. Researchers and companies are actively improving model efficiency by developing smaller, task-specific alternatives to resource-intensive language models. Additionally, leading tech firms are committing to renewable energy for their data centers. Also, many tech companies like AWS, Google and Microsoft are adopting carbon-aware computing to further enhance sustainability by scheduling energy-intensive processes when and where cleaner energy sources are most available.
Beyond energy savings, DTs offer transformative value beyond energy savings, fostering healthier, more resilient, and equitable urban spaces. By enhancing indoor air quality, optimizing energy use, and improving building performance, DTs contribute to occupant comfort, reduce utility costs, and combat energy poverty—addressing key issues that impact well-being today. They also enhance safety and resilience, mitigating risks associated with structural failures and poor performance. Moreover, DTs support the long-term sustainability of buildings, enabling efficient lifecycle management and promoting adaptive reuse to reduce the built environment’s carbon footprint. This holistic approach not only meets current needs but also ensures adaptable and resource-efficient spaces for future generations, building a foundation for a more sustainable and equitable future.
Disclaimer: the applicability of the numerical investigation presented in this post is limited to the underlying assumptions made by the author and the referenced sources. It doesn’t represent a comprehensive analysis of the entire landscape of AI models and building applications. However, it is intended to provide a qualitative assessment of the subject’s controversy.
References / Used Sources
https://www.planradar.com/sa-en/how-ai-changing-construction-industry/
https://www.eea.europa.eu/en/analysis/indicators/greenhouse-gas-emissions-from-energy
https://oneclicklca.com/en/resources/articles/early-stage-decarbonization-anz
https://www.linkedin.com/pulse/how-ai-digital-twins-drive-sustainable-innovation
https://www.electronicshub.org/the-carbon-emissions-of-big-tech/
https://query.prod.cms.rt.microsoft.com/cms/api/am/binary/RW1lMjE
https://www.gstatic.com/gumdrop/sustainability/google-2024-environmental-report.pdf
https://www.weforum.org/stories/2024/07/generative-ai-energy-emissions/
https://www.wired.com/story/ai-energy-demands-water-impact-internet-hyper-consumption-era/
https://news.climate.columbia.edu/2023/06/09/ais-growing-carbon-footprint/
https://carboncredits.com/how-big-is-the-co2-footprint-of-ai-models-chatgpts-emissions/
https://sustainabilitymag.com/articles/digital-twins-predicting-the-future-of-climate-change
https://aws.amazon.com/blogs/iot/sustainability-with-digital-twins/
https://www.renewablematter.eu/en/what-are-digital-twins-and-how-they-can-help-sustainability
https://foundtech.me/digital-twins-and-the-reduction-of-carbon-footprint/?lang=en
Author: Mohamed Khalil, Student of MBA Sustainability Management Class 2 (2024-2026)